NL2Type: Inferring JavaScript Function Types from Natural Language Information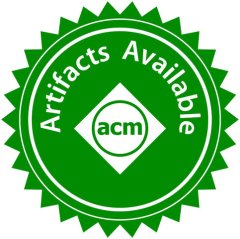
Technical Track
JavaScript is dynamically typed and hence lacks the type safety of statically typed languages, leading to suboptimal IDE support, difficult to understand APIs, and unexpected runtime behavior. Several gradual type systems have been proposed, e.g., Flow and TypeScript, but they rely on developers to annotate code with types. This paper presents NL2Type, a learning-based approach for predicting likely type signatures of JavaScript functions. The key idea is to exploit natural language information in source code, such as comments, function names, and parameter names, a rich source of knowledge that is typically ignored by type inference algorithms. We formulate the problem of predicting types as a classification problem and train a recurrent, LSTM-based neural model that, after learning from an annotated code base, predicts function types for unannotated code. We evaluate the approach with a corpus of 162,673 JavaScript files from real-world projects. NL2Type predicts types with a precision of 84.1% and a recall of 78.9% when considering only the top-most suggestion, and with a precision of 95.5% and a recall of 89.6% when considering the top-5 suggestions. The approach clearly outperforms JSNice, a state-of-the-art approach that analyzes implementations of functions instead of natural language information, and DeepTyper, a recent type prediction approach that is also based on deep learning. Beyond predicting types, NL2Type serves as a consistency checker for existing type annotations. We show that it discovers 39 inconsistencies that deserve developer attention (from a manual analysis of 50 warnings), most of which are due to incorrect type annotations.
Slides (icse2019_NL2Type_slides.pdf) | 454KiB |
Wed 29 MayDisplayed time zone: Eastern Time (US & Canada) change
16:00 - 18:00 | Program Comprehension and ReusePapers / Journal-First Papers / Technical Track at St-Paul / Ste-Catherine Chair(s): Baishakhi Ray Columbia University, New York | ||
16:00 20mTalk | Active Inductive Logic Programming for Code Search Technical Track Aishwarya Sivaraman University of California, Los Angeles, Tianyi Zhang University of California, Los Angeles, Guy Van den Broeck University of California, Los Angeles, Miryung Kim University of California, Los Angeles Pre-print | ||
16:20 10mTalk | The State of Empirical Evaluation in Static Feature LocationJournal-First Journal-First Papers Abdul Razzaq , Asanka Wasala University of Limerick, Chris Exton University of Limerick, Jim Buckley Lero - The Irish Software Research Centre and University of Limerick | ||
16:30 10mTalk | Automatic and accurate expansion of abbreviations in parametersJournal-First Journal-First Papers Yanjie Jiang Beijing Institute of Technology, Hui Liu Beijing Institute of Technology, Jiaqi Zhu Beijing Institute of Technology, Lu Zhang Peking University | ||
16:40 20mTalk | NL2Type: Inferring JavaScript Function Types from Natural Language Information Technical Track Rabee Sohail Malik TU Darmstadt, Jibesh Patra Technical University of Darmstadt, Michael Pradel University of Stuttgart Pre-print Media Attached File Attached | ||
17:00 20mTalk | Analyzing and Supporting Adaptation of Online Code Examples Technical Track Tianyi Zhang University of California, Los Angeles, Di Yang University of California at Irvine, USA, Crista Lopes , Miryung Kim University of California, Los Angeles Pre-print | ||
17:20 20mTalk | DockerizeMe: Automatic Inference of Environment Dependencies for Python Code Snippets Technical Track | ||
17:40 20mTalk | Discussion Period Papers |